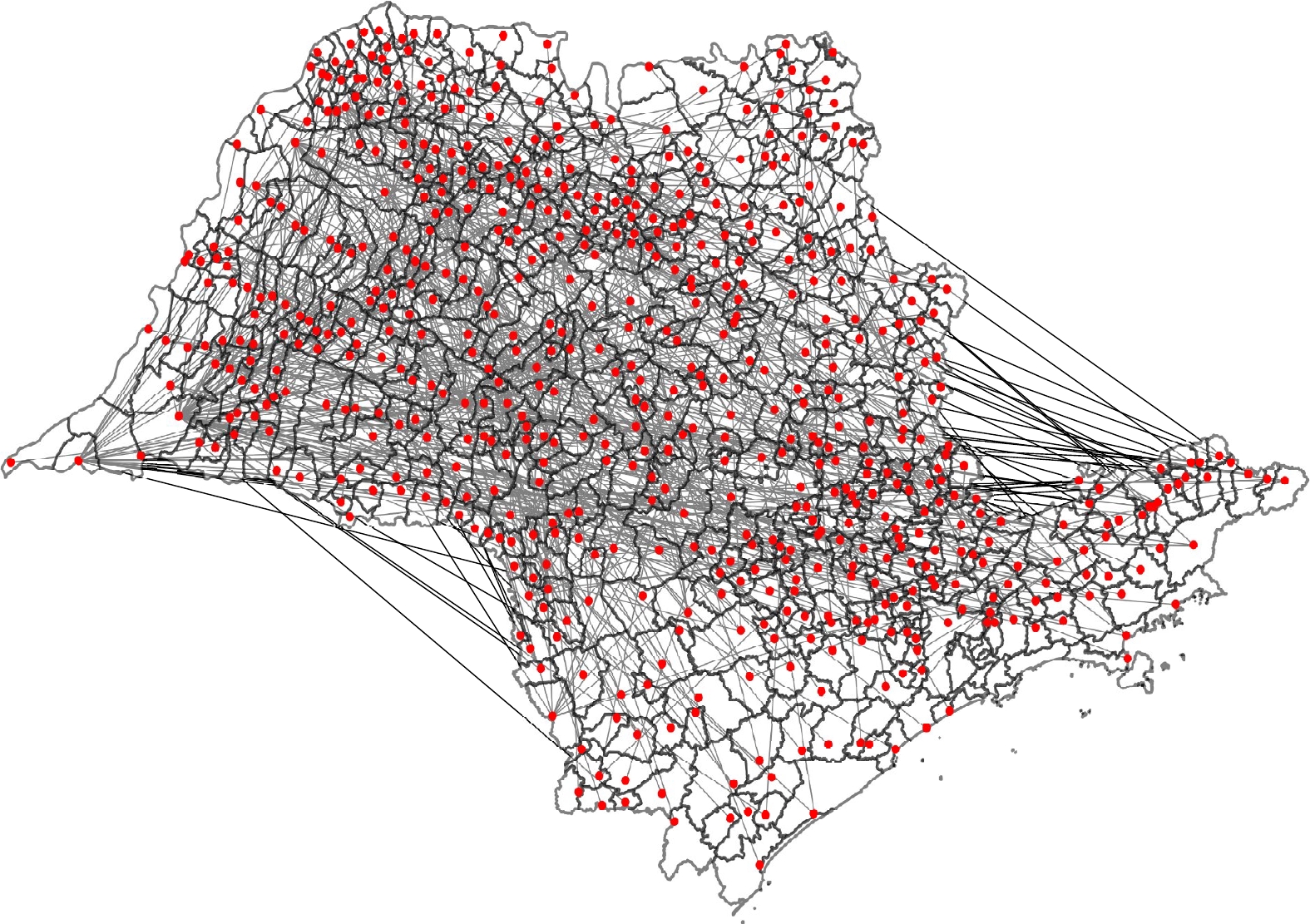
Downloads
Download


This work is licensed under a Creative Commons Attribution 4.0 International License.
Article
Individual-Based Modelling of Animal Brucellosis Spread with the Use of Complex Networks
E. R. Pinto 1, E. G. Nepomuceno 2,*, and A. S. L. O. Campanharo 1
1 Department of Biostatistics, Institute of Biosciences São Paulo State University, Botucatu, São Paulo 18618-970, Brazil
2 Department of Electronic Engineering and Centre for Ocean Energy Research, Maynooth University, Maynooth, Ireland
* Correspondence: erivelton.nepomuceno@mu.ie
Received: 12 October 2022
Accepted: 23 November 2022
Published: 22 December 2022
Abstract: The principal purpose of this work was to study the spread of brucellosis in the state of São Paulo with the help of the complex network theory and to propose control measures for its eradication. For this, the scale-free model of complex networks, widely known in the literature, was used. The effect of vaccination was verified in each of the municipalities in the state of São Paulo and it was observed that when heterogeneity is not taken into account, vaccination becomes ineffective for the eradication of the disease.
Keywords:
Complex networks Optimal Control Brucelosis1. Introduction
Bovine brucellosis is one of the most important infectious and contagious zoonotic disease that hinders livestock productivity and consequently causes major economic losses to producers [1-3]. It is a cause of great concern in places with important agricultural activities, such as in Central and South American countries. Due to this scenario, several countries have already adopted official control programs, with the purpose of reducing impacts caused both on human and animal health. The Ministry of Agriculture, Livestock and Supply (MAPA) of Brazil instituted in 2001 a national program to efficiently fight these two diseases [4]. Therefore, strategies that can assist in understanding the dissemination and prevalence of bovine brucellosis are becoming increasingly necessary. An approach that has been successful in this regard is mathematical modelling, which allows the purpose of strategies to understand the epidemiological impact of a vaccine on a herd [4-8]. Mathematical modelling is commonly used in epidemiology through a compartmental approach, with the use of compartmental model (Susceptible Infected Recovered SIR).
In particular, for the bovine brucellosis several contributions have been published through a compartmental approach using ordinary differential equations [4, 9, 10], Individual/Agent Based Model [11, 12] and theory of complex networks [13-15]. Two of these contributions have drawn attention, as they are two essential techniques in reducing the prevalence of bovine brucellosis. The first one, proposed by Souza et al. [4] is an adaptation of Amaku [16] which uses the combination of two vaccines (B19 and RB51) for a significant decrease of bovine brucellosis. The second one, proposed by Lentz [17] which looks at how infectious diseases can spread through the livestock trade. Although these two articles describe significant advances in brucellosis modelling, Souza [4] has only considered a population of cattle with a fixed percentage of vaccination, and Lentz [17] has investigated the spread of brucellosis in a trade network and does not consider vaccination. According to [18] uniform vaccination is ineffective for the eradication of diseases in heterogeneous populations. Therefore, it is necessary to consider the heterogeneity of the cattle trade and the individual vaccination of each municipality. To satisfy these needs, in this work a combination of a compartmental model with complex networks was used to write the dynamics of bovine brucellosis in herds in the state of São Paulo.
The compartmental models and complex network theory have been increasingly employed in modelling infectious processes [19, 20]. Recent studies have shown that complex networks are a natural support for the study of the spread of infectious diseases in humans and animals [21-23]. We can cite some examples of the use of real animal networks in the spread of diseases, such as in [24], where the authors have used location of animals with high temporal and spatial resolution. The authors in [25] have studied the combination of movements of cattle and sheep, while authors in [26] have combined the livestock movement network with a layer of spatial contact between the wards.
In this work, the optimal vaccination was investigated considering each city in the state of São Paulo as a single entity. The state of São Paulo can be seen as a good case study, as it presents substantial complexity and enough data to carry out our investigation. This state presents a dimension similar to some European countries, such as Spain. In addition, parameters and real data from herds in the state of São Paulo (Brazil) were used. The results show that the heterogeneity of connections has a strong influence on the spread of brucellosis and that when we assume only one optimal vaccination for the entire state, it is ineffective. The application of engineering and control theory techniques has brought some light on epidemiological problems and shown that such problems are relevant. In our previous work [27], optimal control has been employed to give some insights in situations where there is no model, or the model is very poor. The control law of optimal control defines a decisive approach of vaccination in the beginning of an epidemic. Our society has been faced with an unprecedented challenge with the Covid-19 (Coronavirus Disease 2019), and works like that show that a quick response is really important to mitigate the effects of the epidemics. Therefore, we hope that this article can increase the awareness for policy-makers in order to distribute the vaccine as much as heterogeneously as possible. The main contribution of the paper was the application of optimal control to show the importance of a heterogeneous vaccination policy in order to improve eradication efficiency. A real network in the Sao Paulo state was used to distinguish a state level uniform vaccination and local (municipal) level of vaccination. We have shown that using the same level of vaccination, same cost, a heterogeneous policy was able to eradicate the disease, different from what was observed in a homogeneous approach.
The rest of this article is organized as follows: after this introduction, section 2 describes the compartmental model used. Complex networks are presented in section 3. Optimal Control is presented in section 4. The results are presented and discussed in section 5.
2. Basic Concepts
2.1. Compartmental Model
A compartmental model was proposed by Souza et al. [4] to simulate the dynamics of brucellosis in a given population of bovine females in the rearing system for production of milk. The population can be divided into six compartmental classes, that is, susceptible females ( ), vaccinated females (
and
), primiparous and multiparous females latents (
and
) and, finally, primiparous and multiparous females infectious (
and
).
Figure 1 shows a flowchart of interactions between bovine females of the classes ,
,
,
,
,
and
. The parameters
,
,
,
,
and
represent mortality, contact, infection, latency, abortion and, birth rates, respectively. It is observed that a
proportion of calves born to infected mothers becomes a latent carrier, a
portion of susceptible individuals is vaccinated with R19 by moving to the vaccinated 1 state and a
portion of susceptible individuals is vaccinated with RB51 by moving to the vaccinated 2 state.
Figure 1. Schematic representation of the compartmental model developed by Souza et al. [4] consisting of susceptible bovine females ( ), primiparous latent (
), primiparous infected (
), multiparous latent (
), multiparous infected (
), vaccinated with R19 (
) and vaccinated with RB51 (
).
From the flowchart shown in Figure 1, brucellosis can be dynamically described by the following set of equations:
where accounts for the total number of bovine females:
. The size of the population is constant
, therefore:
Because of the ease with which domestic animals can be transported and traded, brucellosis has spread over long distances. The interactional nature of the brucellosis prevalence in multiple regions calls for in-depth discussions. As a result, the patch model was used in this study to describe brucellosis between distinct regions, more specifically the model considers the immigration and emigration of animals among n patches (see Figure 2).
Figure 2. Schematic diagram of cattle migration. Red solid line represents the migration and cattle emigration among patches.
By rewriting our system, we get:
with,
where with
represents immigration rate of cattles from the
th patch to the
th patch. The variable
denotes emigration rate of the cattles. They satisfy the following relationship in (9).
2.2. Complex Networks
A complex network is described by a set of
vertices
and a set of
edges
, where
. In several real networks, edges are associated with weights to represent distances, capacity, traffic or some other measure of connection between two vertices, in this case, the network is represented by
, where
constitutes a set of weights of the
edges [28].
A network can be expressed mathematically by an adjacency matrix , where if two vertices
and
are connected, the position
will be equal to 1 representing presence of an edge; otherwise, it will be zero. In a network with weight
, the position
will be equal to the weight of the edge that connects
and
[29]. Recent investigations have presented the theory of complex networks as a natural support for the investigation of disease propagation [30], contributing to the study of structural characteristics and helping to better understand their implications. Many complex networks models have been suggested in the literature with the aim of reproducing patterns of connections found in real networks [28].
In this work, the scale-free network model proposed by Barabási and Albert [31] was used to investigate the spread of bovine brucellosis in the state of São Paulo, as it can generate networks with structures more similar to those of real.
3. Optimal Control
An optimal control was proposed to reduce sick cattle and the percentage of vaccinated cattle. For this, you want to minimize the value of the following performance index:
where, is the final time,
is the number of cities,
and
are weights associated with priorities that you want to minimize (
),
and
are the B19 and RB51 vaccines, respectively. The desired optimal control pair
is expressed as
where represents the set of all Lebesgue measurable control variables expressed by
3.1. Pontryagin's Maximum Principle
Necessary conditions for optimal control variables and state variables are described as follows. By introducing adjoin functions, the Pontryagin maximum principle was utilized, and it represents an optimal control in terms of the state and adjoint functions. The minimization problem (10) is assigned to a Hamiltonian of minimization like the following:
where are adjoin functions corresponding to
and
, respectively. Optimality conditions for the control problem are supported by the following equations according to Pontryagin maximum principle:
Taking into account a bounded optimal control problem with and
, condition (14) provides the optimal control pair
as follows
Condition (15) establishes the state system (3) together with its initial values. Additional requirement (16) produces the following adjoin system:
where,
The adjoint system (18) ought to satisfy the so-called transversality condition because we assume a free terminal time for this control problem.
As a result, the optimal control represents the optimality condition for the existence of the optimal solution. (17), the state system (3) and adjoin system (18), which are simultaneously solved. Due to the closed and convex admissible control and performance index integrand (10) is also convex in and
,
, then control (17) and minimize (10). It means that the Pontryagin maximum principle defines optimality in terms of both necessary and sufficient conditions.
3.2. Numerical Solution
For a model with no control, i.e., , it results in a system without adjoin system. Thus, we applied a forward-in-time iterative technique over state system (3) using initial conditions
. We used the forward-backward sweep method based on the fourth-order Runge-Kutta algorithm as in, however, for a model with control, the optimality conditions include a set of differential equations with initial conditions and another set with terminal conditions[32]:
(a) Define the initial guess for control variables and
;
(b) Solve the initial value problem of state system (3) using forward-in-time;
(c) Find the solution of the backward-in-time the terminal value problem of adjoin system (18);
(d) Compute the new control values (17) using previous results. The update uses the average between current and past values.;
(e) Calculate performance index;
(f) Iterate the process until desired tolerance.
The numerical solution is well established and it works fine for well-behaved functions. It is important to know that some knowledge about the systems is required to establish the initial guess. The forward and backward methods are solved by standard numerical algorithms and two scenarios (global and local) have been designed to test its solution.
4. Results
According to the 2017 Agricultural Census, the state of São Paulo (SP) has a herd of about 8.3 million cattle [33]. For this study, the database provided by the Brazilian Institute for Geography and Statistics (IBGE) was used in relation to the sizes of cattle in each of the 619 municipalities in the state of São Paulo[33]. The trade connections between the herds were simulated in order to try to reproduce real networks. According to [34], the distribution of connections in a cattle movement network follows a power law. Therefore, the scale-free network of Barabási and Albert [31] was used, where the hubs are the cities that have the largest herds. Figure 3 shows the cattle trade network in the state of São Paulo, in which the vertices represent the city and the edges represent the trade between them.
Figure 3. Livestock movement network in the state of São Paulo in 2017. Vertices represent the city and the edges represent the trade between them.
After the trade network was defined, two different scenarios for the spread of brucellosis were analyzed. More specifically, in the first scenario, the state of São Paulo was considered as a single unit, generating only optimal control, that is, an equal vaccination was considered for all municipalities in the state. In the second scenario, optimal control was calculated for each of the municipalities in the state of São Paulo, generating 619 optimal control in total.
Figure 4 shows the and
vaccination rates as a function of time, where the brown lines represent the vaccination in each of the 619 cities and the black line represents the average for the state. For the simulations, the following parameters were used:
,
,
,
,
,
,
,
,
,
,
,
,
,
,
with
and
. Futhermore
,
,
,
,
,
,
and
.
Figure 4. Vaccination rates and
as a function of time for each of the cities (brown lines) and the average of vaccination rates (black lines).
Figure 5 shows the comparison of the evolution of brucellosis assuming the optimal control for each city (black lines) and the average of the optimal control (red lines). It can be observed that when we do not consider the heterogeneity of connections and thus adopt only the optimal control for the entire state, the disease will still persist in the population. However, if we assume different control for each of the cities, the disease will be eradicated.
Figure 5. Evolution of brucellosis propagation using the optimal control for each city (black lines) and the average of the optimal controls (red lines).
5. Conclusion
In this work, a compartmental model for the spread of brucellosis in conjunction with complex networks was computationally implemented. Afterwards, the optimal control over the compartmental model was proposed using Pontryagin's maximum principle, more specifically, a reduction of sick cattle and the number of vaccinated cattle was proposed. Two different scenarios for the spread of brucellosis in the state of São Paulo were analyzed, in the first scenario only the common optimal control was considered, that is, homogeneous vaccination for all municipalities in the state of São Paulo and in the second scenario different optimal control were considered, observing the connections and sizes of each one of the municipalities, thus creating a heterogeneous vaccination. It was observed that when the heterogeneity of size and connections is not taken into account, optimal vaccination becomes ineffective in eradicating the disease.
It is concluded that the evolution of a given disease, in particularly, the brucellosis, is highly sensitive to the network topology used, and that homogeneous vaccination is not a good strategy for brucellosis eradication. Therefore, it is extremely important to identify the animal transit networks in order to propose effective control measures such as vaccination and slaughter. An important issue that has not been investigated in this work is related to the dynamics of the topology of the network. Future work should study mechanisms to incorporate changes in the topology according to different connections made among farms. We also intend to elaborate an epidemiological model for the dissemination of this disease in the state of São Paulo using the combination of complex networks with a stochastic model, such as the Individual Based Model. The results would be possible to be compared with real data.
Author Contributions: E.G. Nepomuceno and A.S.L.O. Campanharo: conceptualization; E.R. Pinto, E.G. Nepomuceno and A.S.L.O. Campanharo: methodology ; E.R. Pinto: software; E.R. Pinto and E.G. Nepomuceno: validation; E.R. Pinto and E.G. Nepomuceno: formal analysis; E.R. Pinto and E.G. Nepomuceno: investigation; A.S.L.O. Campanharo and E.G. Nepomuceno: resources; E.R. Pinto: data curation; E.R. Pinto: writing-original draft preparation; E.G. Nepomuceno: writing-review and editing; E.R. Pinto:visualization; E.G. Nepomuceno and A.S.L.O. Campanharo: supervision; A.S.L.O. Campanharo and E.G. Nepomuceno: project administration; E.G. Nepomuceno and A.S.L.O. Campanharo: funding acquisition.
Funding: This research received no external funding.
Conflicts of Interest: The authors declare no conflict of interest.
Acknowledgments: A. S. L. O. Campanharo was supported by São Paulo Research Foundation (grant 2018/25358-9). E. R. Pinto was supported by CAPES (Brazil) - Finance Code 001. Core 2 duo computer with 4Gb of RAM, on the Linux operating system, has been used to perform all simulations. Codes were written in C and figures were elaborated with XmGrace.
References
- Paulin, L.M.; Ferreira Neto, J. S, A experiência Brasileira no combate à Brucelose bovina. Arq. Inst. Biol., 2002, 69: 105−112.
- Pappas, G.; Papadimitriou, P.; Akritidis, N.;, et al, The new global map of human brucellosis. Lancet Infect. Dis., 2006, 6: 91−99.
- Franc, K.A.; Krecek, R.C.; Häsler, B.N.;, et al, Brucellosis remains a neglected disease in the developing world: A call for interdisciplinary action. BMC Public Health, 2018, 18: 125.
- de Souza, V.A.F.; Ferreira Neto, J.S.; Amaku, M.;, et al, Mathematical modeling of bovine brucellosis control using the RB51 vaccine. Semin. Ciênc. Agrár., 2016, 37: 3767−3776.
- Borba, M.R.; Stevenson, M.A.; Gonçalves, V.S.P.;, et al, Prevalence and risk-mapping of bovine brucellosis in Maranhão state, Brazil. Prev. Vet. Med., 2013, 110: 169−176.
- Inchaisri, C.; Prasomsri, P.; Boonserm, T.;, et al, A stochastic simulation model for brucellosis eradication in goat flocks in an area with high flock prevalence but low animal prevalence. Small Rumin. Res., 2016, 136: 227−237.
- H ou, Q.; Zhang, F, Global dynamics of a general brucellosis model with discrete delay. J. Appl. Anal. Comput., 2016, 6: 227−241.
- de Alencar Mota, A.L.A.; Ferreira, F.; Ferreira Neto, J.S.;, et al, Large-scale study of herd-level risk factors for bovine brucellosis in Brazil. Acta Trop., 2016, 164: 226−232.
- Abatih, E.; Ron, L.; Speybroeck, N.;, et al, Mathematical analysis of the transmission dynamics of brucellosis among bison. Math. Methods Appl. Sci., 2015, 38: 3818−3832.
- L i, M.T.; Pei, X.; Zhang, J.;, et al, Asymptotic analysis of endemic equilibrium to a brucellosis model. Math. Biosci. Eng., 2019, 16: 5836−5850.
- Havas, K.A.; Boone, R.B.; Hill, A.E.;, et al, A brucellosis disease control strategy for the kakheti region of the country of Georgia: An agent-based model. Zoonoses Public Health, 2014, 61: 260−270.
- Nepomuceno, E.G.; Barbosa, A.M.; Silva, M.X.;, et al, Individual-based modelling and control of bovine brucellosis. R. Soc. Open Sci., 2018, 5: 180200.
- R oy, S.; McElwain, T.F.; Wan, Y, A network control theory approach to modeling and optimal control of zoonoses: Case study of brucellosis transmission in Sub-Saharan Africa. PLoS Negl. Trop. Dis., 2011, 5: e1259.
- Cipullo, R.I.; Grisi-Filho, J.H.H.; Dias, R.A.;, et al, Cattle movement network, herd size, and bovine brucellosis in the state of Mato Grosso, Brazil. Semin. Ciênc. Agrár., 2016, 37: 3777−3792.
- Darbon, A.; Valdano, E.; Poletto, C.;, et al, Network-based assessment of the vulnerability of Italian regions to bovine brucellosis. Prev. Vet. Med., 2018, 158: 25−34.
- Amaku, M.; Dias, R.A.; Ferreira Neto, J.S.;, et al, Modelagem matemática do controle de Brucelose bovina por vacinação. Arq. Bras. Med. Vet. Zootec., 2009, 61: 135−141.
- Lentz, H.H.K.; Koher, A.; Hövel, P.;, et al, Disease spread through animal movements: A static and temporal network analysis of pig trade in Germany. PLoS One, 2016, 11: e0155196.
- Wa ng, Z.; Moreno, Y.; Boccaletti, S.;, et al, Vaccination and epidemics in networked populations—an introduction. Chaos Solitons Fractals, 2017, 103: 177−183.
- Jin, Z.; Li, S.P.; Zhang, X.G.; et al. Epidemiological modeling on complex networks. In
Complex Systems and Networks ; Lü, J.H.; Yu, X.H.; Chen, G.R.; et al., Eds.; Springer: Berlin/Heidelberg, 2016; pp. 51–77. doi: 10.1007/978-3-662-47824-0_3 - Biegus, T.; Kwasnicka, H. Complex networks in the epidemic modelling. In
9th Asian Conference on Intelligent Information and Database Systems ,Kanazawa ,Japan ,April 3–5 ,2017 ; Springer: Kanazawa, Japan, 2017; pp. 202–213. doi: 10.1007/978-3-319-54472-4_20 - Campanharo, A.S.L.O.; Ramos, F. M, Hurst exponent estimation of self-affine time series using quantile graphs. Phys. A, 2016, 444: 43−48.
- Liljeros, F.; Edling, C.R.; Amaral, L.A.N.;, et al, The web of human sexual contacts. Nature, 2001, 411: 907−908.
- Kupennan, M.; Abramson, G. Small world effect in an epidemiological model. In
The Structure and Dynamics of Networks ; Newman, M.; Barabási, A.L.; Watts, D.J., Eds.; Princeton University Press: Princeton, 2011; pp. 489–492. doi: 10.1515/9781400841356.489 - Ch en, S.; White, B.J.; Sanderson, M.W.;, et al, Highly dynamic animal contact network and implications on disease transmission. Sci. Rep., 2014, 4: 4472.
- Ruget, A.S.; Rossi, G.; Pepler, P.T.;, et al, Multi-species temporal network of livestock movements for disease spread. Appl. Netw. Sci., 2021, 6: 15.
- Chaters, G.L.; Johnson, P.C.D.; Cleaveland, S.;, et al, Analysing livestock network data for infectious disease control: An argument for routine data collection in emerging economies. Philos. Trans. R. Soc. B Biol. Sci., 2019, 374: 2180264.
- Nepomuceno, E.G.; Peixoto, M.L.C.; Lacerda, M.J.;, et al, Application of optimal control of infectious diseases in a model-free scenario. SN Comput. Sci., 2021, 2: 405.
- Newman, M.E.J.
Networks: An Introduction . Oxford University Press: New York, 2010. doi: 10.1093/acprof:oso/9780199206650.001.0001 - da F Costa, L.; Rodrigues, F.A.; Travieso, G.;, et al, Characterization of complex networks: A survey of measurements. Adv. Phys., 2007, 56: 167−242.
- Pinto, E.R.; Nepomuceno, E.G.; Campanharo, A.S.L.O, Impact of network topology on the spread of infectious diseases. Trends Comput. Appl. Math., 2020, 21: 95−115.
- Barabási, A.L.; Albert, R, Emergence of scaling in random networks. Science, 1999, 286: 509−512.
- Bakhtiar, T, Optimal intervention strategies for cholera outbreak by education and chlorination. IOP Conf. Ser.: Earth Environ. Sci., 2016, 31: 012022.
- Instituto Brasileiro de Geografia e Estatística.
Censo Agropecuário 2017: Resultados Definitivos ; IBGE: Rio de Janeiro, 2019. Available online: https://loja.ibge.gov.br/censo-agropecuario-2017-resultados-definitivos.html(accessed on 5 November 2022). - Bigras-Poulin, M.; Thompson, R.A.; Chriel, M.;, et al, Network analysis of Danish cattle industry trade patterns as an evaluation of risk potential for disease spread. Prev. Vet. Med., 2006, 76: 11−39.